Google's Datacenters on Punch Cards
If all digital data were stored on punch cards, how big would Google's data warehouse be?
James Zetlen
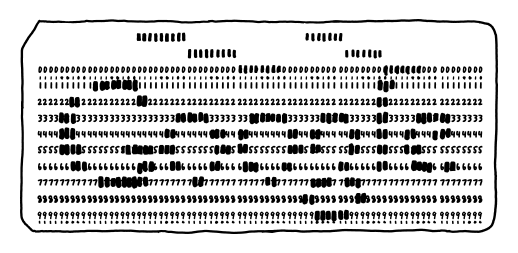
Google almost certainly has more data storage capacity than any other organization on Earth.
Google is very secretive about its operations, so it's hard to say for sure. There are only a handful of organizations who might plausibly potentially have more storage capacity or a larger server infrastructure. Here's my short list of the top contenders:
Honorable mentions:
- Amazon (They're huge, but probably not as big as Google.)
- Facebook (They're on the right scale and growing fast, but still playing catch-up.)
- Microsoft (They have a million servers,[1]Data Center Knowledge: [Ballmer: Microsoft has 1 Million Servers although no one seems sure why.)
Let's take a closer look at Google's computing platform. try to figure out how much computing power Google has.
Follow the money
We'll start by following the money. Google's aggregate capital expenditures–spending on building stuff stuff—adds up to somewhere over $12 billion dollars. [2]I'm excluding the cost of an extremely expensive building they bought in New York. —adds up to somewhere over $12 billion dollars.[3]Data Center Knowledge: Google’s Data Center Building Boom Continues: $1.6 Billion Investment in 3 Months Their biggest data centers cost half a billion to a billion billion, dollars, so they can't have more than 20 or so of those.
On their website,[4]Data center locations Google acknowledges that they have datacenters in the following locations:
- Berkeley County, South Carolina
- Council Bluffs, Iowa
- Atlanta, Georgia
- Mayes County, Oklahoma
- Lenoir, North Carolina
- The Dalles, Oregon
- Hong Kong
- Singapore
- Taiwan
- Hamina, Finland
- St Ghislain, Belgium
- Dublin, Ireland
- Quilicura, Chile
In addition, they appear to operate a number of other large datacenters (sometimes through subsidiary corporations), including:
- Eemshaven, Netherlands
- Groningen, Netherlands
- Budapest, Hungary
- Wrocław, Poland
- Reston, Virginia
- Additional sites near Atlanta, Georgia
They also operate equipment at dozens to hundreds of smaller locations around the world.
Follow the power
To figure out how many servers Google is running, we can look at their electricity consumption. power bill. Unfortunately, we can't just sneak up to a datacenter and read the meter.[5]Actually, wait, can we? Somebody should try that. this. Instead, we have to do some digging.
The company disclosed that in 2010 they consumed an average of 258 megawatts of power.[6]Google used 2,259,998 MWh of electricity in 2010, which translates to an average of 258 megawatts. How many computers can they run with that?
We know that their datacenters are quite efficient, only spending 10-20% of their power on cooling and other overhead.[7]Google: Efficiency: How we do it To get an idea of how much power each server uses, we can look at their "container data center" concept from 2005. It's not clear whether they actually use these containers in practice—it may just have been a now-outdated experiment—but it gives an idea of what they consider(ed) reasonable power consumption. The answer: 215 watts per server.
Judging from that number, in 2010, they were operating around a million servers.
They've grown a lot since then. By the end of 2013, the total amount of money they've pumped into their datacenters will be three or four times what it was as of 2010. They've contracted to buy over three hundred megawatts of power at just three sites,[8]Google: Purchasing clean energy which is more than they used for all their operations in 2010.
Based on datacenter power usage and spending estimates, my guess would be that Google is currently running—or will soon be running—between 1.8 and 2.4 million servers.
But what do these "servers" actually represent? Google could be experimenting in all kinds of wild ways, running boards with 100 cores or 100 attached disks. If we assume that each server has a couple[9]Anywhere from 2 to 5 of 2 TB disks attached, we come up with close to 10 exabytes [10]As a refresher, the order is: kilo, mega, giga, tera, peta, exa, zetta, yotta. An exabyte is a million terabytes. of active storage attached to running clusters.
10 Exabytes
The commercial hard disk industry ships about 8 exabytes worth of drives annually. [12] [10] IDC: Worldwide External Disk Storage Systems Factory Revenue Declines for the Second Consecutive Quarter Those numbers don't necessarily necessarilly include companies like Google, but in any case, it seems likely that Google is a large piece of the global hard drive market.
To make things worse, given the huge number of drives they manage, Google has a hard drive die every few minutes.[11]Eduardo Pinheiro, Wolf-Dietrich Weber and Luiz Andre Barroso, [Failure Trends in a Large Disk Drive Population This isn't actually all that expensive a problem, in the grand scheme of things—they just get good at replacing drives—but it's weird to think that when a Googler runs a piece of code, they know that by the time it finishes executing, one of the machines it was running on will probably have suffered a drive failure.
Google tape storage
Of course, that only covers storage attached to running servers. What about "cold" storage? Who knows how much data Google—or anyone else—has stored in basement archives?
In a 2011 phone interview with Paul Mah of SMB Tech, Simon Anderson of Tandberg Data let slip [13] [11] SMB Tech: Is Tape Still Relevant for SMBs? that Google is the world's biggest single consumer of magnetic tape cartridges, purchasing 200,000 per year. Assuming they've stepped up their purchasing since then as they've expanded, this could add up to another few exabytes of tape archives.
All this could
Putting it all together
Let's assume Google has a storage capacity of 15 exabytes, [12]As a refresher, the order is: kilo, mega, giga, tera, peta, exa, zetta, yotta. An exabyte is a million terabytes. or 15,000,000,000,000,000,000 bytes.
A punch card can hold about 80 characters, and a box of cards holds 2000 cards:
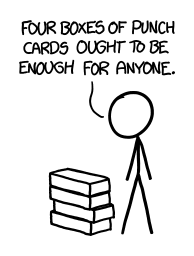
15 exabytes of punch cards would be enough to cover my home region, New England, to a depth of about 4.5 kilometers. That's three times deeper than the ice sheets that covered the region during the last advance of the glaciers:
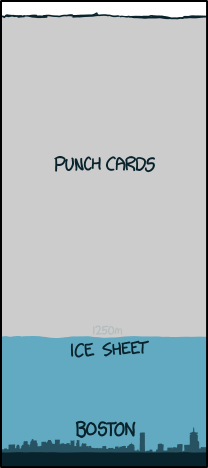
That seems like a lot.
However, it's nothing compared to the ridiculous claims by some news reports about the NSA datacenter in Utah.
NSA datacenter
The NSA is building a datacenter in Utah. Media reports claimed that it could hold up to a yottabyte of data, [14] [13] CNET: NSA to store yottabytes in Utah data centre which is patently absurd.
Later reports changed their minds, suggesting that the facility could only hold on the order of 3-12 exabytes. [15] [14] Forbes: Blueprints Of NSA's Ridiculously Expensive Data Center In Utah Suggest It Holds Less Info Than Thought We also know the facility uses about 65 megawatts of power, [16] [15] Salt-Lake City Tribune: NSA Bluffdale Center won’t gobble up Utah’s power supply which is about what a large Google datacenter consumes.
A few headlines, rather than going with one estimate or the other, announced that the facility could hold "between an exabyte and a yottabyte" of data [17] [16] Dailykos: Utah Data Center stores data between 1 exabyte and 1 yottabyte ... which is a little like saying "eyewitnesses report that the snake was "the escaped snake is believed to be between 1 millimeter and 1 kilometer long."

Uncovering further Google secrets
There are a lot of tricks for digging up information about Google's operations. Ironically, many of them involve using Google itself—from Googling for job postings in strange cities to using image search to find leaked cell camera photos of datacenter visits.
However, the best trick for locating secret Google facilities might be the one revealed by ex-Googler talentlessclown on reddit: [18] [17] reddit: Can r/Australia help find Google's Sydney data center? Seems like a bit of a mystery...
The easiest way to find manned Google data centres is to ask taxi drivers and pizza delivery people.
There's something pleasing poetic about that. Google has created what might be the most sophisticated information-gathering apparatus in the history of the Earth ... and the only people with the Earth ... yet the people with the most information about them are the pizza delivery drivers.
Who watches the watchers?
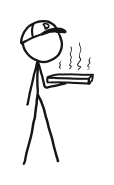
Apparently, Domino's.
Apparently, Domino's.